What are 2D and 3D multispectral maps, and why are they so valuable?
The 2D and 3D multispectral maps are an advanced way of capturing data beyond human vision, offering detailed views of Earth’s surface. Specialized cameras collect images across various wavelengths, including infrared and near-infrared, revealing details invisible to the naked eye. These details, like healthy vegetation or heat variations, are captured because each spectral band targets specific elements on the ground. Through sophisticated processes like aerial data collection and digital modeling, multispectral maps have become powerful tools for science, resource management, and policy. They bridge the gap between what we see and hidden patterns in our environment, representing a significant technological leap in understanding our planet. The key advantage is a multilayered perspective, offering insights invisible to the naked eye. This powerful technology is essential for many fields. In agriculture, it helps farmers monitor crop health and use water more efficiently. For environmental conservation, it allows us to track deforestation and protect our forests. In urban planning, it helps identify areas that trap heat and guide sustainable development.
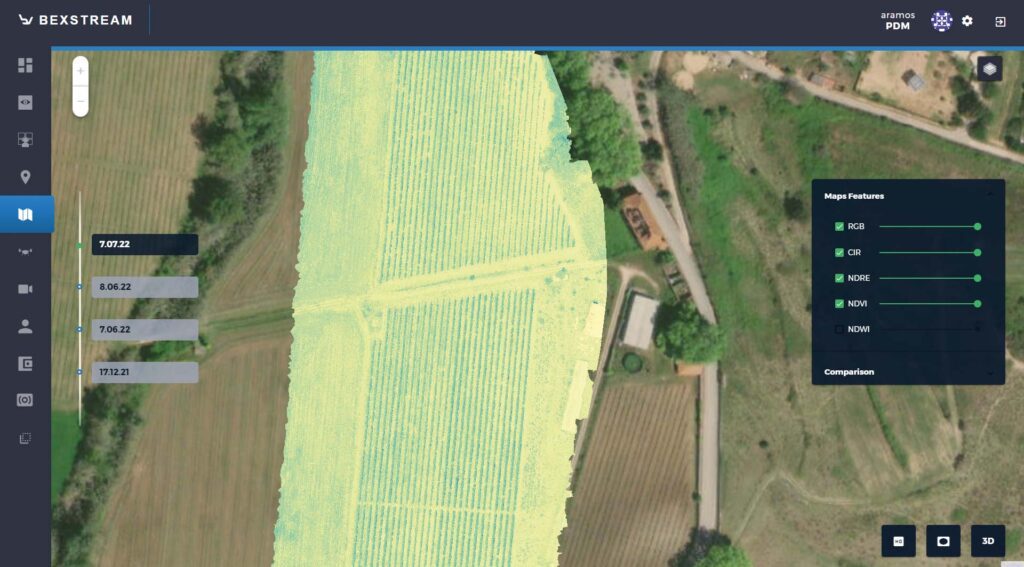
Prior efforts, limitations and evolution in photogrammetry & remote sensing
The field of photogrammetry and remote sensing has long been instrumental in mapping and analyzing the Earth’s surface. Traditional methods predominantly relied on direct photographic techniques and basic satellite imagery to gather spatial information. Existing methods laid the groundwork for environmental applications, but they struggle to capture the intricate details needed for in-depth analysis. This hinders our ability to fully understand complex environmental issues.
Advancements in Methodologies: SfM and MVS
- Spectral Limimation - Conventional photographic methods primarily capture images within the visible spectrum, limiting the range of detectable environmental variables. Many crucial signs of environmental health, like plant stress, become clearer when we look beyond visible light. These signs are often revealed in non-visible wavelengths, such as infrared or ultraviolet, which our eyes can't detect.
- Spatial Resolution - Earlier satellite missions frequently provided satellite imagery with low spatial resolution, posing challenges in detecting fine-scale environmental changes. This limitation hinders our ability to actively detect subtle environmental changes. It also makes it difficult to precisely map small features. These capabilities are essential for both detailed environmental management and scientific research.
- Temporal Resolution - The frequency at which traditional satellite images are captured can be insufficient for monitoring rapid environmental changes. This delay can hinder timely analysis and response to events such as natural disasters, seasonal changes, or fast-paced urban development.
- Dimensionality - Direct photographic methods and basic satellite imagery are limited in their ability to capture the three-dimensional structure of landscapes and objects. This limitation constrains the depth of analysis possible, particularly in applications like forestry, urban planning, and geomorphological studies.
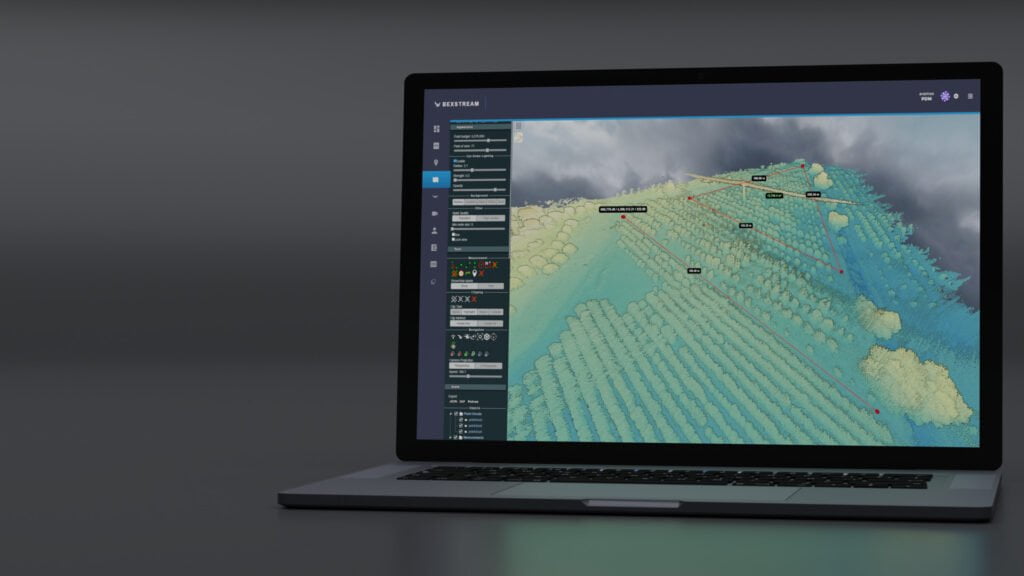
Limitations of Traditional Methods
Limitations of traditional methods were recognized. The scientific community responded by adopting SfM and MVS – more sophisticated methodologies. These advancements deliver substantial improvements in the quality and usefulness of spatial data.
- Structure from Motion (SfM) - SfM is a photogrammetric technique that constructs three-dimensional structures from two-dimensional image sequences. By analyzing multiple photographs taken from different viewpoints, SfM algorithms can deduce the three-dimensional coordinates of points on the surface being photographed. This method not only enhances spatial resolution but also introduces the dimensionality missing in traditional methods, allowing for the creation of detailed 3D models of the environment.
- Multi-View Stereopsis (MVS) - Building on the principles of SfM, Multi-View Stereopsis further refines the process of generating 3D models by using images captured from multiple angles to reconstruct a scene. MVS techniques focus on analyzing the disparity between images taken from different viewpoints to estimate depth, significantly improving the accuracy and detail of the 3D models produced.
Both SfM and MVS represent significant advancements in our ability to generate high-resolution, accurate, and multi-dimensional maps and models of the Earth’s surface. New methodologies overcome many limitations of traditional photogrammetry and remote sensing techniques. This advancement opens up new possibilities for in-depth environmental analysis. Empowered by these methods, scientists and researchers can now explore and understand the complex dynamics of natural and built environments. The result? Unprecedented clarity and detail are revealed.
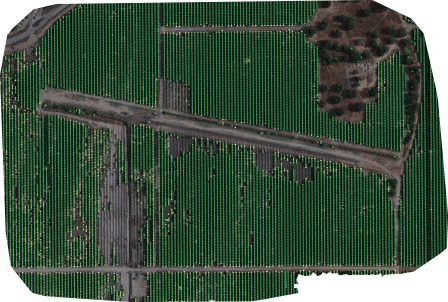
Methodology of generating multispectral maps
Generating 2D and 3D multispectral maps relies on a carefully structured methodology. This method first involves a series of procedures. These procedures seamlessly integrate advanced imaging techniques with sophisticated data processing. Ultimately, this process translates aerial imagery into detailed, multidimensional representations of the Earth’s surface. Below are the steps:
Data Load/Input
Airborne platforms equipped with imaging sensors collect aerial images. These images form the crucial first step in creating 2D and 3D multispectral maps, which capture Earth’s surface in detail. This stage is instrumental in gathering raw data, which forms the basis for all subsequent analysis and modeling.
Structure from Motion (SfM)
The transition from the Data Load/Input phase to the Structure from Motion (SfM) step in the creation of multispectral maps is a seamless progression that hinges on the preparation and systematic organization of the collected aerial imagery. This transition is facilitated through a series of interconnected actions and analyses that prepare the dataset for complex 3D modeling.
- Metadata Extraction - This is the initial step of SfM, where relevant metadata (e.g., GPS coordinates, camera orientation) is extracted from each image. This information is crucial for accurate positioning and orientation in the subsequent reconstruction process.
- Feature Detection - Algorithms are employed to detect distinct visual features within the images, such as edges or specific textures, which are pivotal for matching and tracking across multiple images.
- Feature Matching - This step involves identifying and pairing similar features detected across different images. Successful feature matching is essential for accurately reconstructing the scene in 3D.
- Track Creation - Here, sequences or "tracks" of matched features across multiple images are developed. These tracks help in understanding the movement and structure of the scene, serving as a guide for the 3D reconstruction process.
- Reconstruction - Using the feature tracks as references, a sparse 3D model of the scene is assembled. This model represents the initial, less dense reconstruction of the scene's geometry.
- Undistort - Prior to densification, any distortions present in the images (typically caused by the camera lens) are corrected. This step ensures that the images accurately reflect the true shapes and sizes of objects in the scene.
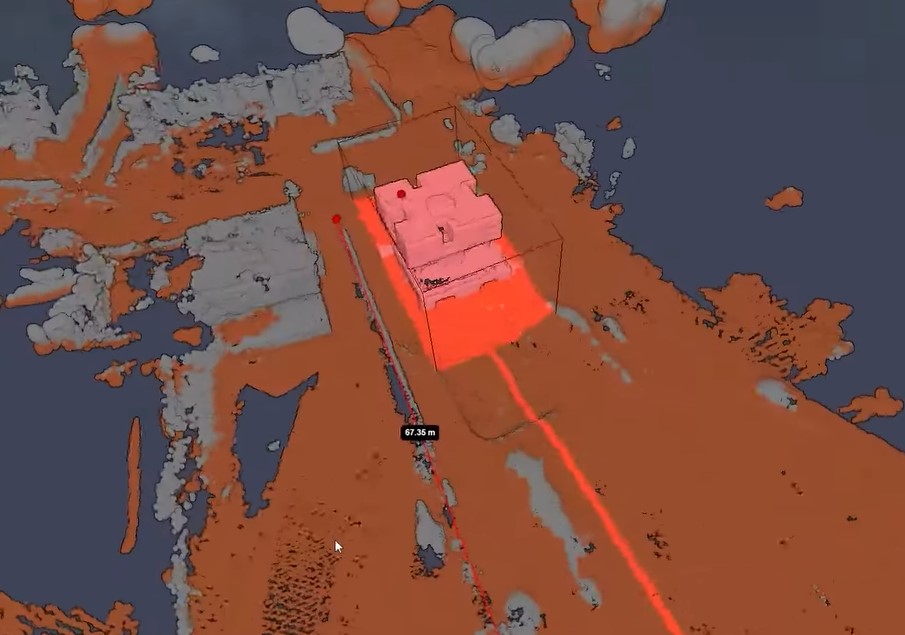
Multi-View Stereo (MVS)
- Stereo Pair Selection - In the MVS process, pairs of images are selected based on their suitability for depth analysis. This selection is guided by the overlap and the angles between images to maximize depth information extraction.
- Depth Map Estimation - For each selected pair (or sets) of images, depth maps are estimated, detailing the distance of surfaces from the camera's perspective.
- Depth Maps Filtering - The estimated depth maps undergo filtering to remove noise and improve accuracy, ensuring that only reliable depth information is retained.
- Depth Map Fusion - The refined depth maps from multiple views are then fused into a single, coherent depth model. This model provides a more detailed and accurate representation of the scene's geometry.
Meshing Reconstruction
Following the MVS process, the detailed transformation from space function definitions through to the extraction of isosurfaces takes place. This step creates a mesh representation of the model, adding surface details and textures to the previously constructed depth model.
The SfM and MVS processes, along with meshing reconstruction, together create a workflow that transforms sets of aerial images into detailed, textured 3D models.This approach combines two types of information to create highly accurate scene reconstructions. It uses geometric data from individual images and depth data obtained by analyzing image pairs.
Meshing Reconstruction
Following the MVS process, the detailed transformation from space function definitions through to the extraction of isosurfaces takes place. This step creates a mesh representation of the model, adding surface details and textures to the previously constructed depth model.
The SfM and MVS processes, along with meshing reconstruction, together create a workflow that transforms sets of aerial images into detailed, textured 3D models. This advanced method combines the shapes from single images with depth data from pairs of pictures. This results in incredibly precise 3D models of the photographed scene.
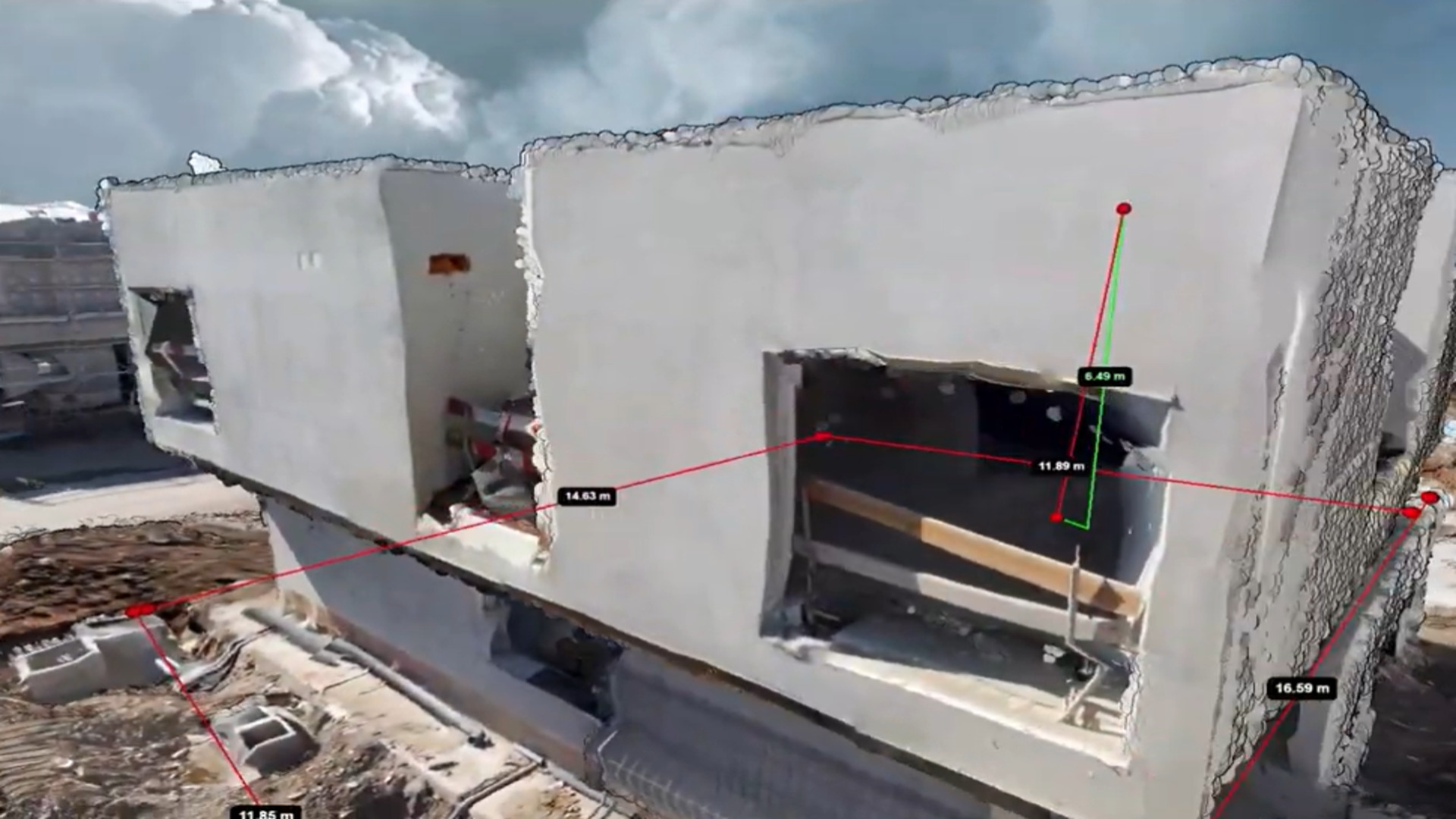
Texturing Reconstruction
After the creation of a 3D model via SfM, MVS, and meshing reconstruction, texturing reconstruction is the process that brings the model to life by adding realistic surface details.
- Preprocessing - After the creation of a 3D model via SfM, MVS, and meshing reconstruction, texturing reconstruction is the process that brings the model to life by adding realistic surface details.
- View Selection -Determines the most suitable images or perspectives for applying textures to the 3D model. This step is crucial for optimizing the visual quality of the model, ensuring textures are applied from angles that minimize distortion and cover the model uniformly.
- Color Adjustment - Fine-tunes the colors of the textures derived from the aerial images to match the real-world appearance of the terrain and features. This step addresses any discrepancies in color that may arise from varying lighting conditions or camera settings during the image capture process, enhancing the realism of the textured model.
Georeferencing
Geo-referencing is the process of aligning the textured 3D model with real-world geographic coordinates, crucial for ensuring the model accurately represents the physical location and orientation of the surveyed area.
Aligning the model ensures it matches real-world locations, making it compatible with other geographic data. This unlocks its potential for practical uses like urban planning, environmental monitoring, and navigation.
Orthomap creation
- Orthomap - Provides aerial view of the surveyed area, flattening the 3D model into a 2D map while maintaining the spatial accuracy of features. This map is useful for a wide range of applications that require accurate, top-down imagery, such as land use planning, asset management, and geographic analysis.
Experimental Results: Practical Applications and Challenges
The outlined method for 2D and 3D multispectral maps, using RGB, multispectral, and thermal data, has shown major improvements in environmental monitoring, agriculture, and urban planning. Applying a comprehensive workflow of data collection, SfM, MVS, texturing, and geo-referencing produced detailed and accurate landscape models. These models serve multiple purposes, from assessing crop health and irrigation needs in agriculture to enhancing studies of urban heat islands and environmental conservation efforts.
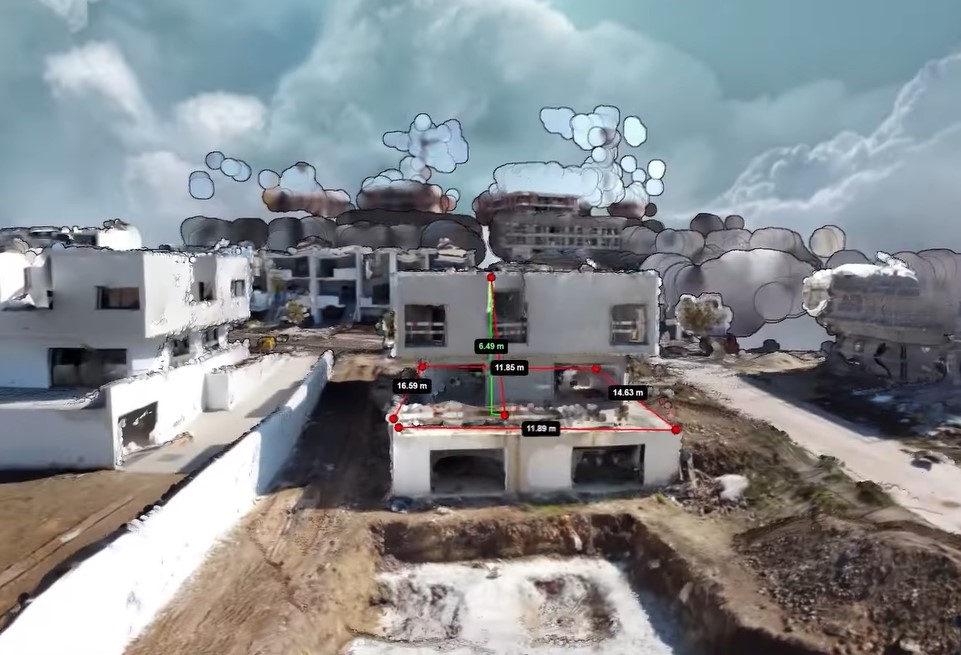
Challenges Encountered and Solutions
We encountered challenges during applying this methodology. These challenges included handling the massive amount of data, ensuring the accuracy of the 3D models, and dealing with the complexities of thermal imaging. Below is an analysis of these challenges and the solutions implemented to address them:
Data Volume and Processing Time:
Challenge: High-resolution aerial images for detailed 2D and 3D maps require a massive amount of data. This puts a strain on computer processing power, resulting in long wait times to create the maps.
Solution: Optimization techniques were applied to streamline data processing, including parallel processing and cloud computing resources. Additionally, algorithms were refined to efficiently manage and process large datasets, effectively reducing the time required for model generation.
Accuracy of 3D Models:
Challenge: Ensuring the spatial accuracy of 3D models, especially in complex environments with diverse terrain and vegetation, proved to be a substantial hurdle.
Solution: Enhanced calibration methods and advanced feature matching algorithms were employed to improve the precision of 3D reconstructions. The integration of ground control points (GCPs) and rigorous post-processing validation also contributed to achieving high levels of model accuracy.
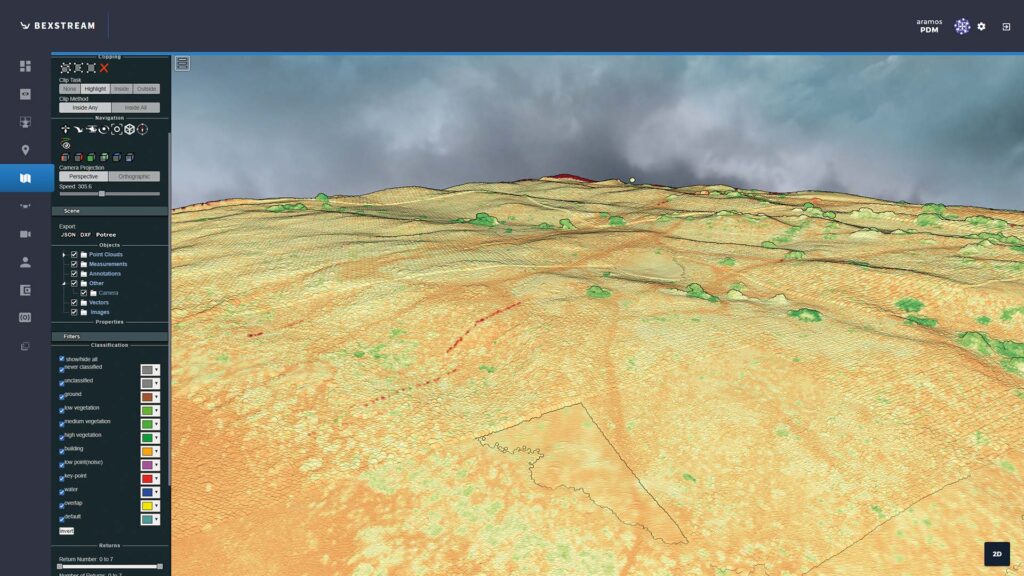
Thermal Imaging Integration:
Challenge: Incorporating thermal imaging into the multispectral mapping process was challenging due to the low contrast and featureless nature of thermal images, making feature detection and matching difficult.
Solution: To improve feature visibility in thermal images, for example as seen in our sustainable vineyard resource management research studies, we developed specialized preprocessing steps. We then adopted a hybrid approach, combining thermal data with RGB and multispectral imagery to maximize the strengths of each type. This approach allowed us to successfully integrate thermal information into multispectral maps, revealing valuable insights into temperature variations and heat sources.
Environmental Conditions and Lighting Variability:
Challenge: Varying lighting conditions and environmental factors such as cloud cover and shadows affected the consistency and quality of the aerial images.
Solution: Strategic planning of data collection missions to coincide with optimal lighting and weather conditions was implemented. Where inconsistencies were unavoidable, advanced image processing techniques, including radiometric calibration and shadow compensation, were applied to normalize the data.
Implications of the Experimental Results
Experiments highlight the power of multispectral maps for understanding our world. This detailed data, acquired through advanced techniques, opens doors for environmental analysis, optimizing agriculture, and sustainable urban planning. By capturing beyond the visible spectrum, these maps reveal crucial insights for monitoring ecosystems, managing water, and planning sustainable cities. The versatility of these maps, captured with beXStream cloud-based drone remote control, underlines their value across various fields, from detecting subtle changes in vegetation to identifying heat signatures in urban areas.
Effectiveness of the Adopted Methodologies
The methodologies employed in this study, particularly those adapted from OpenDroneMap’s workflow, have proven to be highly effective in generating accurate and detailed multispectral maps. The integration of Structure from Motion (SfM) and Multi-View Stereo (MVS) techniques, alongside advanced texturing and georeferencing processes, facilitated the creation of high-resolution 3D models from aerial imagery. The successful application of these methodologies underscores the importance of a comprehensive and integrated approach to data processing in remote sensing. By leveraging OpenDroneMap’s open-source tools, the study benefited from a robust and community-supported platform, enabling efficient processing of large datasets and the production of highly detailed spatial models. This validation of OpenDroneMap’s workflow within the context of multispectral mapping highlights its potential as a valuable tool for researchers and professionals in the field.
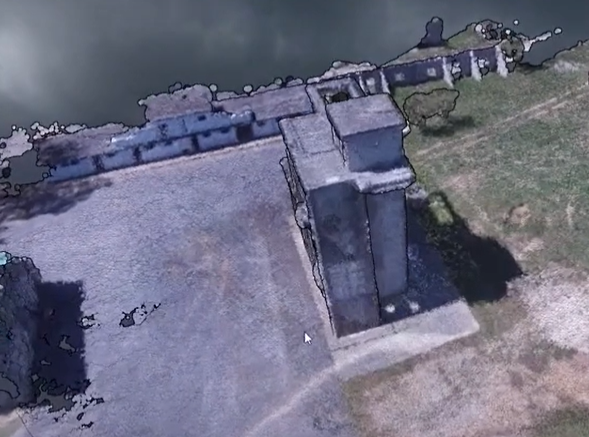
Future Directions for Research and Application
The field of aerial multispectral mapping is poised for breakthroughs. Future research could refine data processing algorithms, particularly for large-scale projects, to boost speed and accuracy of model generation. Moreover, integrating machine learning and AI could offer new methods for feature detection and classification, potentially automating parts of the process and revealing hidden patterns. Additionally, exploring diverse data sources like LiDAR and SAR data could enhance the multidimensional analysis capabilities of 2D and 3D multispectral maps. On the application side, multispectral mapping holds vast potential to contribute to climate change research, disaster response planning, and biodiversity conservation, among other pressing global challenges. As technology and methodologies evolve, so too will their applications, promising richer insights and more effective solutions for various environmental and societal issues.
Key Points
-
Importance of Collision Avoidance
- Multispectral maps are advanced tools that capture data across multiple wavelengths, revealing intricate details about the Earth's surface invisible to the naked eye.
- Their value lies in applications like agricultural management, environmental conservation, and urban planning. -
Limitations of Traditional Photogrammetry & Remote Sensing
- Traditional methods were limited by spectral, spatial, and temporal resolution, and dimensionality, affecting the depth of environmental analysis.
-
Advancements in Methodologies - SfM and MVS
- Structure from Motion (SfM) and Multi-View Stereopsis (MVS) methodologies offer significant improvements in generating high-resolution, accurate 3D models. -
Methodology of Generating Multispectral Maps
- The process involves data collection, SfM for 3D modeling, MVS for depth analysis, meshing reconstruction for creating a mesh representation, and texturing reconstruction for adding surface details. -
Experimental Results & Challenges
- Applications in environmental monitoring, agriculture, and urban planning were highlighted, alongside challenges such as data volume, model accuracy, thermal imaging integration, and environmental condition variability.. -
Implications of Experimental Results
- Multispectral maps enhance understanding of the physical world, with versatile applications across various fields. -
Effectiveness of Adopted Methodologies
- Methodologies, especially those adapted from OpenDroneMap, proved effective in producing detailed multispectral maps. -
Future Directions for Research and Application
- Potential areas include refining data processing algorithms, integrating machine learning, and expanding the use of diverse data sources for broader applications.
Related Questions
Multispectral maps capture data across multiple wavelengths, revealing details about crop health, soil moisture, and irrigation needs. This information makes them invaluable for agriculture, allowing farmers to precisely monitor crops and optimize practices. Learn more about precision farming.
Traditional remote sensing methods were limited by their spectral, spatial, and temporal resolution, along with a lack of dimensionality. These limitations restricted the depth and accuracy of environmental analyses, making it difficult to capture the nuanced details necessary for comprehensive studies. beXStream cloud platform makes it easy for data acquisition and analysis.
Structure from Motion (SfM) and Multi-View Stereopsis (MVS) significantly improve the resolution and accuracy of 3D models by analyzing multiple photographs from different viewpoints. This allows for the detailed reconstruction of the Earth’s surface in three dimensions, capturing intricate details that were previously unattainable.
Generating a multispectral map involves several key steps: starting with the collection of aerial images, followed by Structure from Motion (SfM) for 3D modeling, Multi-View Stereo (MVS) for depth analysis, meshing reconstruction to create a mesh representation, and finally, texturing reconstruction to add realistic surface details to the model.
The main challenges in integrating thermal imaging included the low contrast and lack of distinct features in thermal images, making feature detection and matching difficult. Researchers addressed these challenges by first developing specialized preprocessing steps. These steps enhanced the visibility of features in the data. Then, they employed a hybrid approach. This approach combined thermal data with RGB and multispectral imagery for better integration.
Multispectral maps contribute significantly to urban planning and environmental conservation by providing detailed insights into vegetation health, water stress, and urban heat islands. This information supports sustainable development practices, biodiversity conservation efforts, and efficient resource management.
Adopting OpenDroneMap’s workflow has positively impacted the production of multispectral maps by offering an efficient, robust, and community-supported platform for processing large datasets. This has enabled the creation of detailed spatial models with enhanced accuracy and resolution.
The future of multispectral mapping is bright! Researchers aim to refine data processing algorithms, integrate machine learning for automated feature detection. Most importantly, it aims to explore diverse data sources like LiDAR and SAR equipped with HEIFU pro or VTOne long range full electric VTOL. These advancements could revolutionize environmental monitoring, disaster response, and climate change research. Imagine richer insights and more effective solutions to global challenges!
- Share this Publication