- Terrain classification is essential for enhancing UAV capabilities, supporting tasks such as autonomous navigation, emergency landings, and precision agriculture.
- A novel approach combines static and dynamic texture analysis with UAV rotor downwash effects to differentiate terrain types, including water, vegetation, asphalt, and sand.
Index
- The Challenge of Multi-Terrain Image Classification
- Innovative Algorithms for AI-Powered Terrain Mapping
- Hardware and Software Integration: The Backbone
- Mapping the Unknown: Dynamic Terrain Classification
- Results That Redefine Possibilities
- Applications and Future Prospects
- Conclusion: A Leap Toward Smarter UAVs
- Glossary
- Summary
- FAQ
The Challenge of Multi-Terrain Image Classification
Traditional terrain classification techniques often rely on static image features and face challenges with dynamic environments. This study highlights gaps in previous research, which typically classified terrain based on single static features, limiting their applicability to complex, mixed-terrain images.
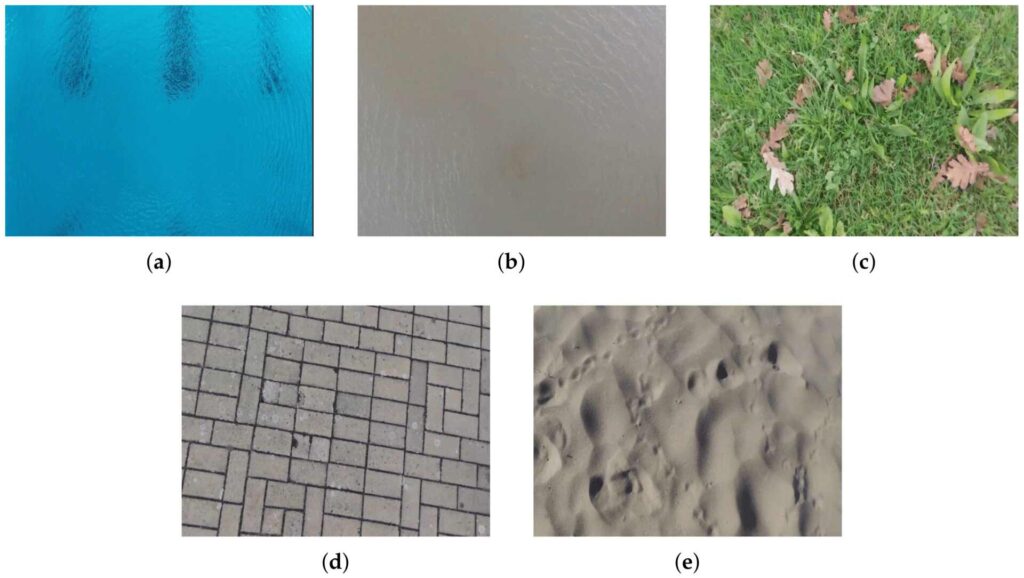
Why Downwash Dynamics Matter
The downwash effect generated by UAV rotors causes significant localised airflow, which interacts with terrain surfaces such as water, vegetation, sand and asphalt. These interactions create distinct movement patterns that are visible in aerial imagery, such as ripples on water or swaying vegetation. Such dynamic changes serve as an additional layer of data, complementing static texture analysis methods like Gray-Level Co-Occurence Matrix (GLCM) or Gray-Level Run Length Matrix (GLRLM). By capturing and analysing these dynamic mapping patterns using algorithms such as optical flow in drone navigation, UAVs can achieve more precise terrain classification, particularly in environments with mixed terrain types or when static texture features alone are insufficient. Incorporating downwash dynamics into terrain classification workflows enhances the system’s ability to differentiate between terrain types, increasing accuracy and robustness in challenging scenarios.
Innovative Algorithms for AI-Powered Terrain Mapping
1. Static Texture Analysis
Gray-Level Co-Occurrence Matrix (GLCM) and Gray-Level Run Length Matrix (GLRLM) analyze pixel intensity relationships to extract features like contrast, homogeneity, and entropy.
2. Dynamic Texture Analysis
Optical Flow Techniques use motion patterns from downwash to identify terrain-specific behaviors, such as circular ripples on water or static responses on asphalt.
3. Neural Network Classifier
Outputs from these algorithms feed into a multilayer perceptron neural network to classify terrains with enhanced accuracy.
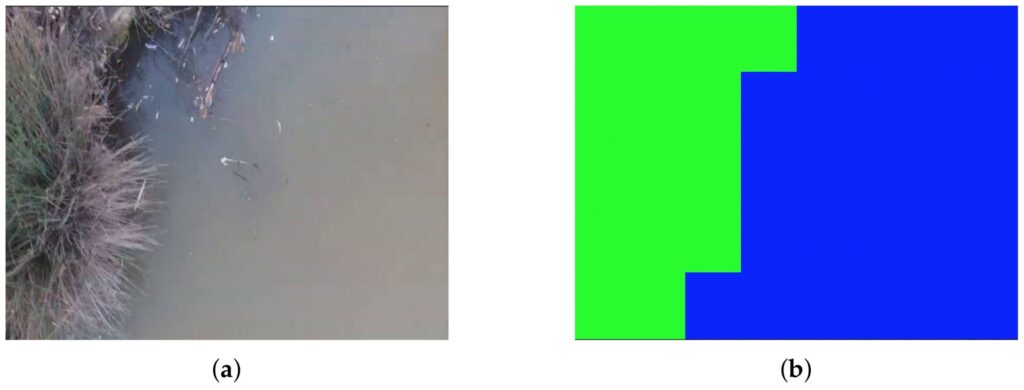
Hardware and Software Integration: The Backbone
The UAV System and Experimental Setup
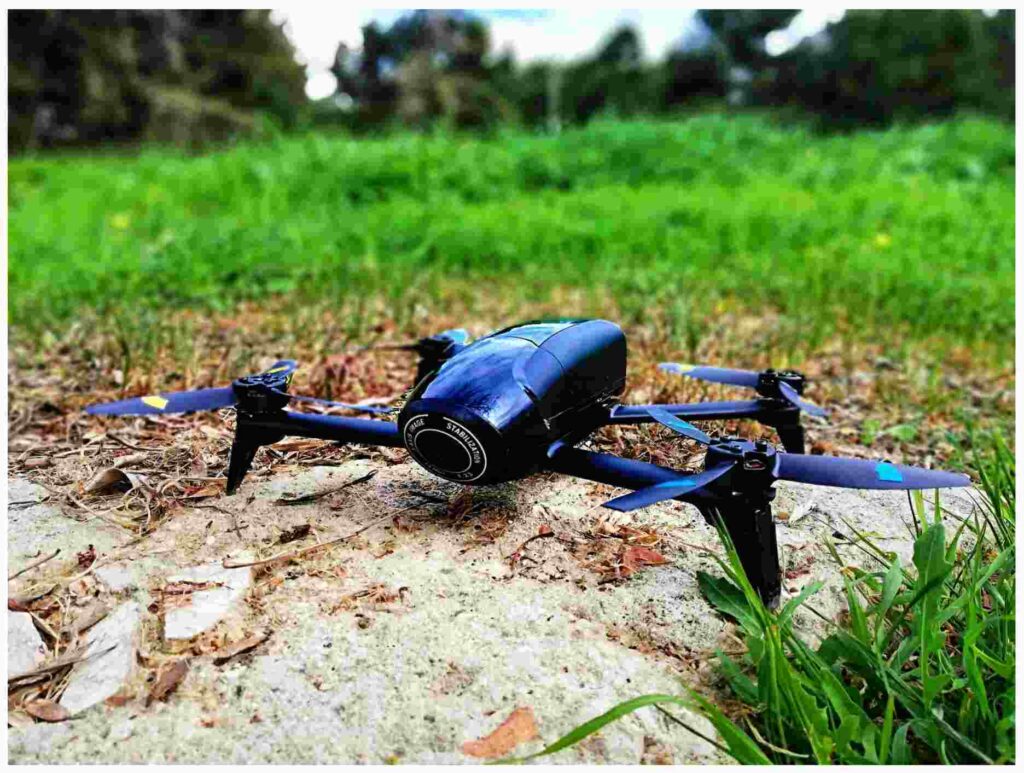
FPGA-Based Acceleration
Mapping the Unknown: Dynamic Terrain Classification
A Real-Time Dynamic Map
Results That Redefine Possibilities
A Real-Time Dynamic Map
The proposed system demonstrates an impressive overall accuracy of 95.14%, surpassing existing methods. Key advantages include:
- Simultaneous classification of multiple terrain types within a single frame.
- High accuracy due to the combination of static and dynamic features.
- Robust performance at low altitudes (1-2 meters), where terrain interaction with downwash is most prominent.
Comparative Analysis
Applications and Future Prospects
A Versatile Tool for Autonomous Systems
The system’s ability to generate real-time, accurate terrain maps opens doors for applications in:
- Autonomous Navigation: Helping surface and ground vehicles avoid obstacles or find paths.
- Precision Agriculture: Enabling detailed crop and soil analysis.
- Rescue Missions: Assisting in identifying safe landing zones or hazardous areas.
Future Enhancements
To further improve the system, the study suggests:
- Expanding capabilities to handle dynamic environments with changing lighting or weather conditions.
- Enhancing obstacle avoidance mechanisms for low-altitude navigation.
- Optimizing performance for higher-resolution imagery without compromising speed.
Conclusion: A Leap Toward Smarter UAVs
This research bridges the gap between static and dynamic terrain classification, paving the way for more intelligent and versatile UAV systems. By integrating cutting-edge algorithms with hardware acceleration and practical mapping tools, it sets a high standard for UAV applications in real-world scenarios.
Glossary
Summary
Key techniques include:
- Static Texture Analysis (GLCM and GLRLM) for features like contrast and entropy.
- Dynamic Texture Analysis (Optical Flow) for motion patterns.
- Neural Network Classifier to combine features and categorize terrains accurately.
Achieving 95.14% accuracy, the system surpasses existing methods and supports applications like navigation, precision agriculture, and rescue missions. Future enhancements include adapting to dynamic lighting, improving obstacle avoidance, and optimizing for high-resolution images. This technology sets a new benchmark for smarter, more versatile UAV systems.
Your Questions Answered
A rapid Q&A about terrain classification for UAVs.
- Gray-Level Co-Occurrence Matrix (GLCM) and Gray-Level Run Length Matrix (GLRLM) for static texture analysis.
- Optical Flow techniques for dynamic texture analysis to detect motion patterns.
- A neural network classifier to combine static and dynamic features for terrain categorization.
It supports applications such as:
- Autonomous navigation for UAVs and ground vehicles.
- Precision agriculture for detailed crop and soil analysis.
- Rescue missions to identify safe zones or hazards.
- Adapting to dynamic lighting and weather changes.
- Enhancing obstacle avoidance mechanisms for low-altitude navigation.
- Optimizing performance for higher-resolution imagery.
- Share this Publication