- UAV testing is becoming increasingly important. However, drone testing often involves either expensive real-world demonstrations or limited, generic 3D simulations.
- The main advantage of this platform is its ability to replicate real-world environments in a virtual setting, making drone testing more realistic, accessible, and scalable.
- Two deep learning algorithms, YOLO v4 and Mask R-CNN, are used to create the 3D simulation environment.
Index
- Current Need for Realistic Simulation Environments
- Comparison: How Existing Simulators Stack Up
- System Architecture and the Use of AI Algorithms
- Key Technologies Behind the Autonomous Environment Generator
- Heifu: the UAV Used in the Simulations
- What’s Next? Improvements to the Autonomous Environment Generator
- Glossary
- 3 Minute Summary
- Your Questions Answered
Current Need for Realistic Simulation Environments
In their paper, Autonomous Environment Generator for UAV-Based Simulation, they propose a novel testbed where machine learning is used to procedurally generate, scale, and place 3D models, resulting in increasingly realistic simulations. Satellite images serve as the foundation for these environments, enabling users to test UAVs in a more detailed representation of real-world scenarios.
Comparison: How Existing Simulators Stack Up
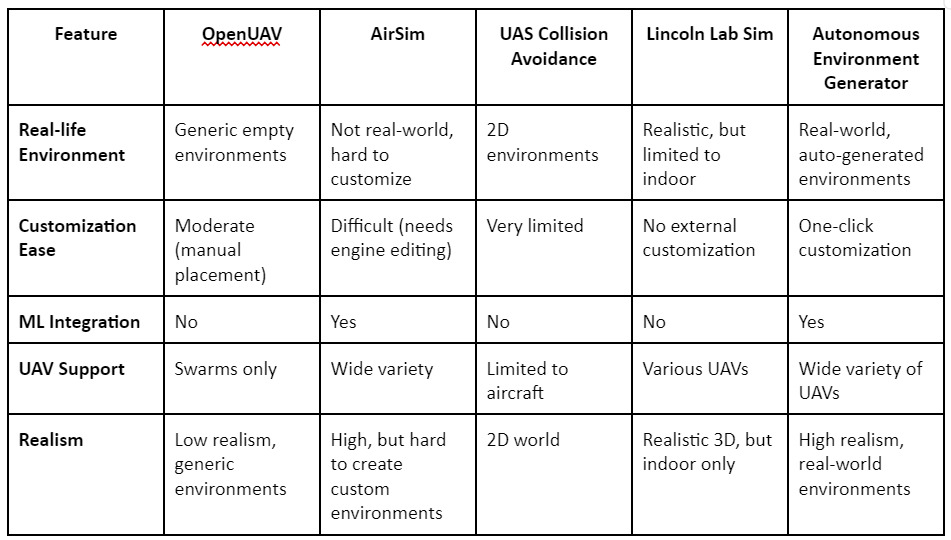
System Architecture and the Use of AI Algorithms
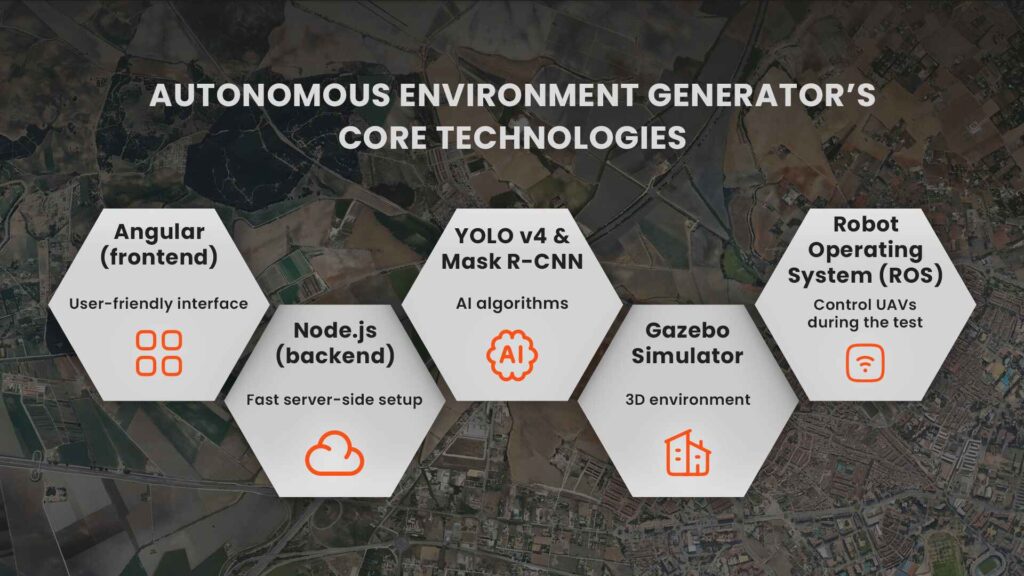
Key Technologies Behind the Autonomous Environment Generator
- Frontend (Angular) - A user-friendly interface that allows users to capture and select satellite images.
- Backend (Node.js) - A fast server-side setup that processes the data and handles real-time communication.
- Gazebo Simulator - A 3D environment that renders the simulation with physical models.
- Robot Operating System (ROS) - enables users to manually or autonomously control UAVs during the test.
Mask R-CNN, on the other hand, is used for road detection which requires pixel-level segmentation. It generates masks that provide more precision for complex shapes that don’t fit neatly into a bounding box. This model was trained using the Spacenet Road Network Detection Challenge dataset.
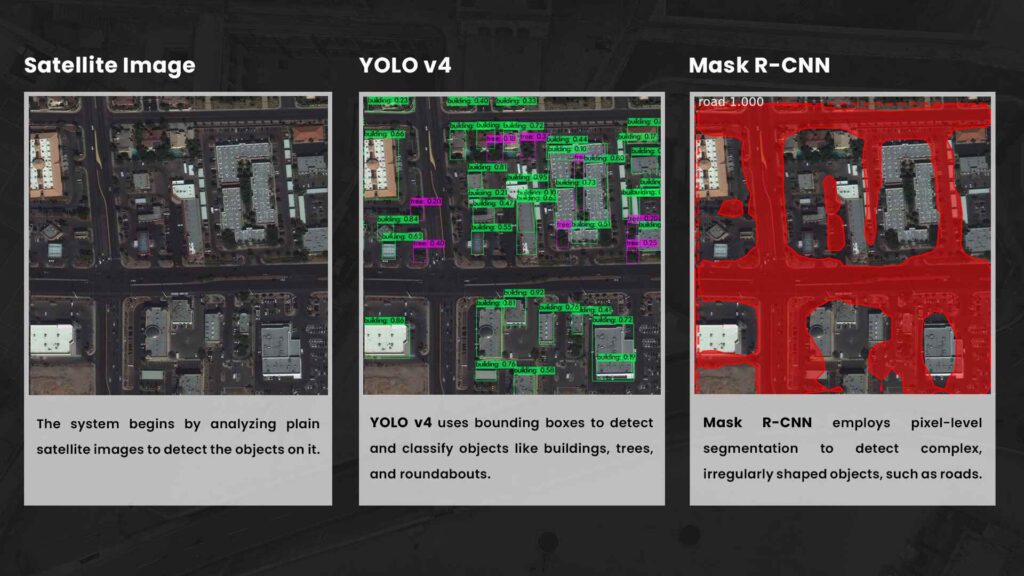
The generator was trained on aerial image datasets and achieves a balance between speed and accuracy. Once the satellite images are processed and objects are detected, the data is sent to the backend, where it is stored for future use. The 3D models of buildings, trees, and other objects are then scaled and placed in the Gazebo simulator at their correct geographic positions. The simulation is controlled using the Robot Operating System (ROS), which enables users to manually or autonomously control UAVs during the test. UAVs interact with these models, allowing testers to simulate real-world flight scenarios, such as navigating through an urban environment.
Both models were tested on a variety of satellite images, and performed well overall. However, some challenges still remain, particularly with the placement of complex building geometries.
What’s Next? Improvements to the Autonomous Environment Generator
- More Object Classes - Expanding the range of detectable objects to include more types of buildings, vegetation, and urban features.
- Faster Load Times - Optimizing the backend processes to reduce load times for large-scale environments.
- Saved Simulations - Developing the ability to reload past simulations and combine different geographic locations for larger test environments.
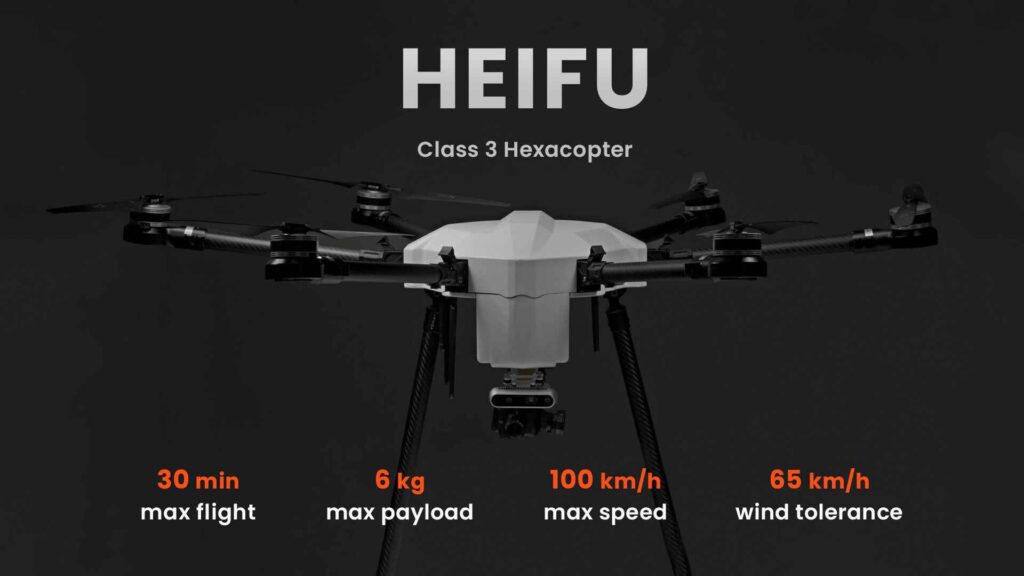
Glossary
Autonomous Environment Generator
YOLO v4 (You Only Look Once, Version 4)
DOTA Dataset
Mask R-CNN
Spacenet Road Network Detection Challenge Dataset
Convolutional Neural Networks (CNNs)
Gazebo 3D Simulator
ROS (Robot Operating System)
3-Minute Summary
By using machine learning algorithms like YOLO v4 and Mask R-CNN, the system can detect objects such as buildings, trees, and roads in satellite images, then place 3D models of these objects into a simulation. The Gazebo simulator is used to render these environments, which can then be navigated by drones controlled via the Robot Operating System (ROS).
The main advantage of this platform is its ability to replicate real-world environments in a virtual setting, making UAV testing more realistic, accessible, and scalable. Current platforms either lack real-world accuracy or require manual placement of 3D objects, but this system automates the process, significantly reducing the time and cost involved in testing.
Despite its success, there are areas for improvement, such as the need for more detailed object models and faster load times. In the future, the platform aims to include the ability to save and reload simulations, making it even more flexible for repeated testing.